๐ฅ2 Million Monthly Downloads๐ฅ
- We are so excited to announce that we surpassed ๐ฎ ๐ ๐ถ๐น๐น๐ถ๐ผ๐ป ๐๐ผ๐๐ป๐น๐ผ๐ฎ๐ฑ๐ per month across Python, Typescript, Java, and Rust. Our community is indexing ๐ฏ๐ถ๐น๐น๐ถ๐ผ๐ป๐ ๐ผ๐ณ ๐๐ฒ๐ฐ๐๐ผ๐ฟ๐ and managing ๐ฝ๐ฒ๐๐ฎ๐ฏ๐๐๐ฒ๐ ๐ผ๐ณ ๐๐ฟ๐ฎ๐ถ๐ป๐ถ๐ป๐ด ๐ฑ๐ฎ๐๐ฎ for AI. It's an open secret that our users have known for some time now - LanceDB is the easiest way to add knowledge to your AI applications and Lance format is the new gold standard for multimodal AI data.
- โค๏ธ We're so grateful for our community, for contributions, feedback, and insights all along the journey. Drop us a line in discord and give us a star on GitHub.
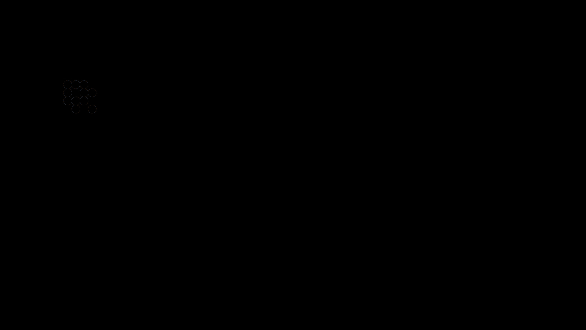
Community contributions
๐ก
A heartfelt thank you to our community contributors of lance and lancedb this past month: @HoKim98 @Jay-ju @SaintBacchus @niyue @FuPeiJiang @MaxPowerWasTaken @emmanuel-ferdman @fzowl @fzliu @umuthopeyildirim@stevensu1977 @gagan-bhullar-tech @kursataktas
๐ก
A shoutout to our community contributor for building a LanceDB Guru on Gurubase. It uses data from LanceDB's Github and documentation to answer questions. ๐ค๐ฌ
https://github.com/lancedb/lancedb/pull/1797
https://github.com/lancedb/lancedb/pull/1797
Good reads
AWS Startup Sr. Solution Architects Kevin Shaffer-Morrison and Giuseppe Battista built a Full Stack Serverless Retrieval Augmented Generation Application on AWS features LanceDB running on Lambda. If a $1000+ monthly bill is not where you want to splurge on, then maybe give this a spin.
Serverless Retrieval Augmented Generation (RAG) on AWS
The year is 2024 and youโre still paying for a vector database when youโre not using it. Not anymore! In this post we explore a fully serverless solution for your Retrieval Augmented Generation (RAG) applications on AWS backed by Amazon Lambda, Amazon Bedrock, Amazon S3, and LanceDB.
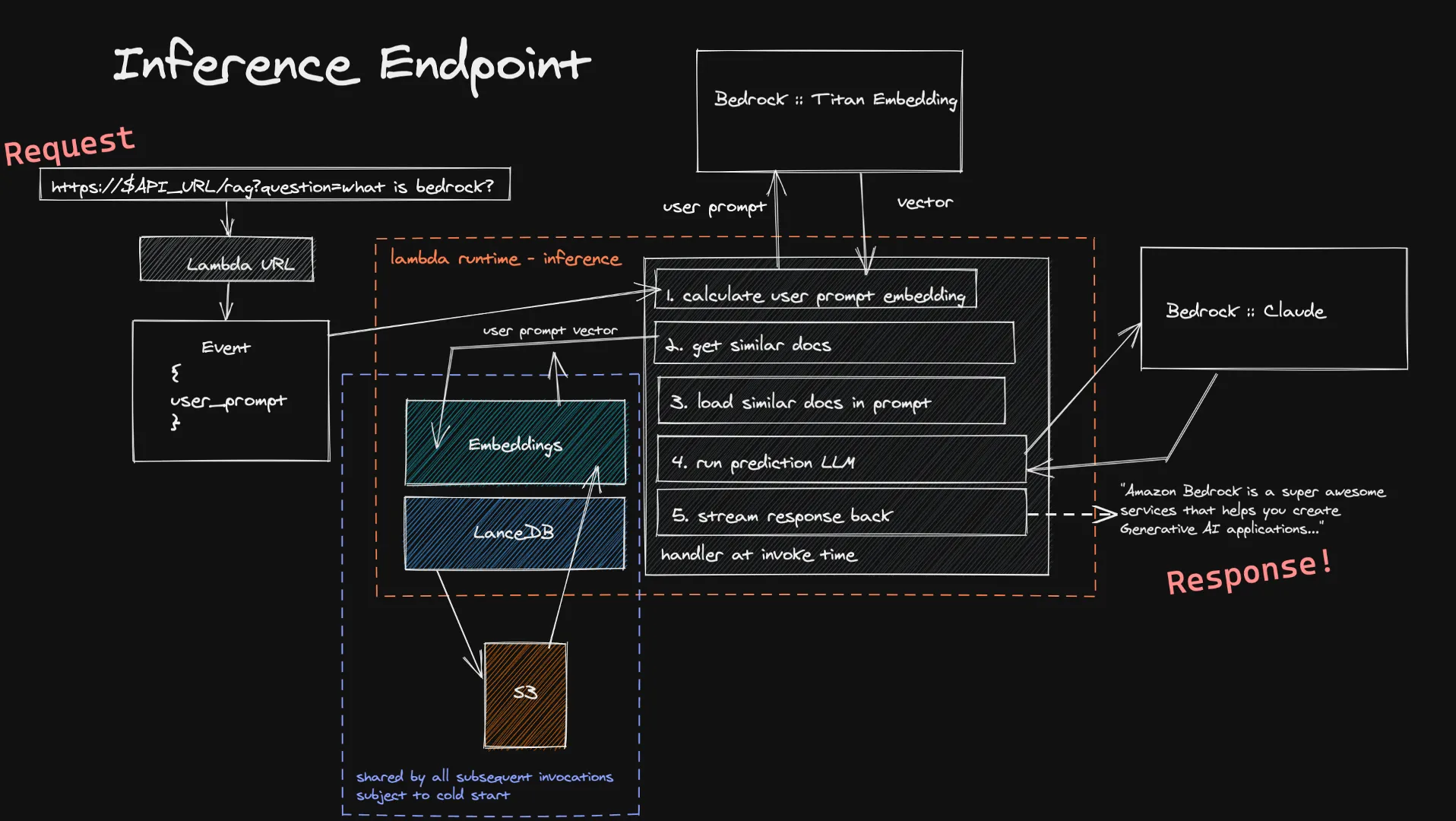
Event recap
LanceDB: Building developer-friendly, multi-modal vector databases
Podcast Episode ยท The Baking Soda Podcast: Featuring Startup Companies on the Rise ยท 11/11/2024 ยท 44m
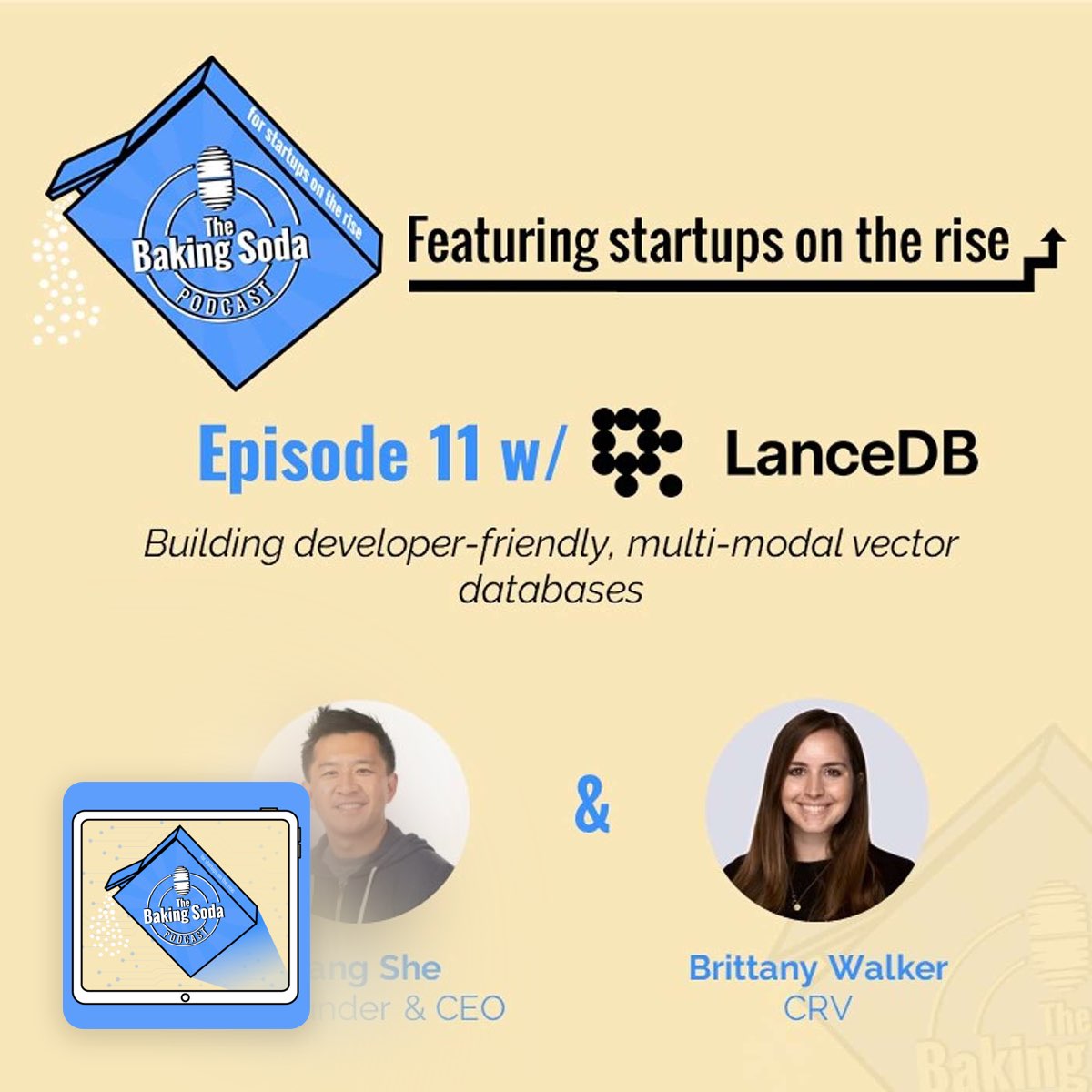
The Baking Soda Podcast on Vector Base, Open Source and AI
Upcoming events
Bridging Big Data and AI: Empowering PySpark with Lance Format for Multi-Modal AI Data Pipelines PyData Global 2024
PySpark has long been a cornerstone of big data processing, excelling in data preparation, analytics, and machine learning tasks within traditional data lake ecosystems. However, the rise of multimodal AI and vector search introduces new challenges that push beyond PySparkโs native capabilities. Sparkโs new Python data source API opens the door for integration with emerging AI data lakes built on the multi-modal Lance format. Lance delivers unparalleled value with its zero-copy schema evolution capability and robust support for large record-size data (e.g., images, tensors, embeddings, etc), simplifying multimodal data storage. Its advanced indexing for semantic and full-text search, combined with rapid random access, enables high-performance AI data analytics to the level of SQL. This powerful combination bridges the gap between traditional big data processing and the demands of modern AI workloads, offering a streamlined approach to handling complex, multi-modal datasets. By unifying PySparkโs robust processing capabilities with Lanceโs AI-optimized storage, data engineers and scientists can efficiently manage and analyze the diverse data types required for cutting-edge AI applications within a familiar big data framework.
Lance Format Office Hours ยท Luma
Welcome to the first Lance Format Community Office Hour!
Event Details
Location: Google Meet (virtual)
https://meet.google.com/akj-vned-gkg
Agenda๏ผ
The LanceDBโฆ
Latest releases
Last month's new releases span across Python, Rust, and Node with following major features and changes:
- Inserting data is now more flexible. Users can omit fields, ignore nullability differences, and provide fields out-of-order.
- Experimental balanced storage added to tackle balancing file sizes when tables have large blob columns.
- Full-text search indices now include unindexed data, just like vector and scalar indexes.
- Several improvements have been made to improve feature parity between Python, Rust, and Node SDKs.
- All LanceDB Cloud clients have been consolidated into a new implementation, providing feature parity between Python, Node, and Rust.
For more details